Harnessing the Power of Labeling Tools for Machine Learning
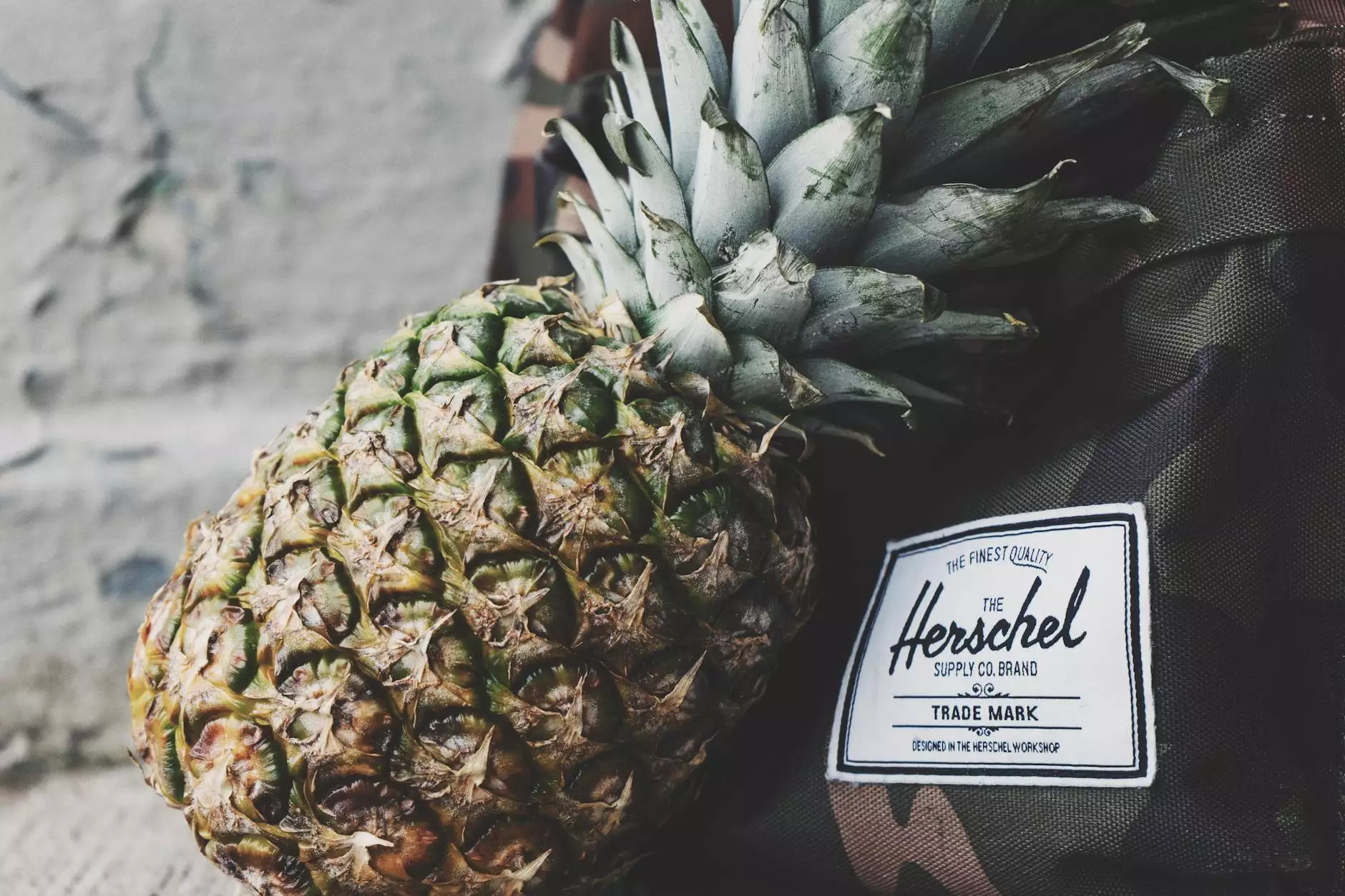
In today's fast-paced technological landscape, the effectiveness of machine learning (ML) largely depends on the quality of data it is trained on. As organizations increasingly turn to sophisticated algorithms and AI-driven solutions, the demand for labeling tools for machine learning has never been higher. These tools play a crucial role in the data annotation process, helping businesses derive meaningful insights from vast datasets. This article delves into the core aspects of these essential tools, their applications, benefits, and how platforms like keylabs.ai can help organizations maximize their data potential.
Understanding Data Annotation
Data annotation involves the process of labeling or assigning tags to data so that AI and ML models can learn from it. This process is indispensable in supervised learning, where models learn from labeled datasets. Proper data annotation allows algorithms to understand the relationship between inputs and outputs, ultimately enhancing their predictive accuracy.
Labeling tools for machine learning enable users to annotate various types of data, including:
- Image Data: Labeled images for object detection, segmentation, and classification tasks.
- Text Data: Annotation of text for natural language processing (NLP) tasks such as sentiment analysis and entity recognition.
- Audio Data: Transcribing and tagging sound files for speech recognition tasks.
- Video Data: Annotating video frames to identify objects, events, or behaviors over time.
The Importance of Labeling Tools
The significance of labeling tools for machine learning cannot be overstated. Here are several reasons why these tools are essential:
1. Enhanced Data Quality
High-quality annotated data is the bedrock of successful machine learning applications. Labeling tools help ensure that data is consistently labeled, which minimizes errors and enhances the learning process.
2. Scalability
As companies scale, the volume of data they need to process also increases. Automated labeling tools can significantly reduce the time and effort required for data annotation, allowing companies to focus on leveraging this data rather than processing it.
3. Cost Efficiency
With the right labeling tools, organizations can reduce the costs associated with manual annotation processes. Automated tools decrease the labor hours required for data preparation, enabling teams to allocate resources more effectively.
4. User-Friendly Interfaces
Many modern labeling tools offer intuitive interfaces that streamline the annotation process. This ease of use empowers data scientists and analysts to quickly annotate data sets without extensive training.
Choosing the Right Labeling Tool for Your Needs
Selecting the appropriate labeling tools for machine learning involves several critical considerations:
1. Type of Data
Different tools cater to various data types. Consider whether you need tools for image, text, audio, or video data annotation to find a suitable solution.
2. Ease of Integration
Evaluate how well a labeling tool integrates with your existing data pipelines. A seamless integration process fosters efficiency and ensures that data flows smoothly between systems.
3. Customization Options
Look for labeling tools that offer customization features to tailor the annotation process to your specific requirements. This flexibility can significantly impact the quality of your labeled data.
4. Collaboration Features
In a collaborative environment, having tools that facilitate teamwork can enhance productivity. Ensure that the labeling platform supports multiple users and offers features for tracking changes and managing tasks.
Key Features of Effective Labeling Tools
When assessing labeling tools for machine learning, consider the following features that contribute to their effectiveness:
- Automated Annotation: Tools that can partially or fully automate the data labeling process can save time and improve productivity.
- Quality Assurance: Features that allow for review, validation, and correction of annotations ensure higher data quality.
- Support for Collaborative Annotation: Multi-user access enables teams to work simultaneously, facilitating faster project completion.
- Analytics and Reporting: Tools that provide insights into the annotation process can help in assessing performance and identifying bottlenecks.
The Role of Keylabs.ai in Data Annotation
At keylabs.ai, we understand the nuances of machine learning and the importance of data quality. Our platform offers a cutting-edge data annotation tool designed to cater to a variety of industries and applications.
Customized Solutions
We offer customizable data annotation solutions that can adapt to your specific project requirements. Whether you need image, text, or video annotation, our tools are built to handle diverse data types efficiently.
Streamlined Workflow
Our platform ensures a streamlined workflow by integrating seamlessly with your existing systems and allowing for easy collaboration among team members. This capability enhances productivity and reduces time-to-market for ML models.
Commitment to Quality
Quality assurance is at the forefront of our processes. Keylabs.ai implements rigorous validation protocols to ensure that all annotated data meets or exceeds industry standards.
Challenges in Data Annotation and How to Overcome Them
While labeling tools for machine learning are invaluable, several challenges can arise during the data annotation process. Understanding these challenges and knowing how to address them can significantly enhance the project's success rate. Here are some common issues:
1. Ambiguity in Labeling
Data can sometimes be ambiguous, leading to inconsistencies in labeling. To combat this, providing clear guidelines and examples for annotators can help ensure that annotations are as accurate as possible.
2. Handling Large Volumes of Data
The sheer volume of data can be overwhelming, making it difficult to annotate everything manually. Automated tools can assist significantly in these situations, allowing businesses to manage larger datasets with ease.
3. Ensuring Consistency Across Annotations
Inconsistencies can arise from different annotators working on the same dataset. To address this, implement a robust quality control process that includes regular audits and feedback sessions with annotators.
4. Training Annotators
A lack of training can lead to poorly annotated data. Investing in training sessions for annotators can ensure they understand the importance of their work and the context of the data being labeled.
Future Trends in Labeling Tools for Machine Learning
The landscape of labeling tools for machine learning is rapidly evolving. Staying informed about the latest trends is crucial for businesses to remain competitive. Some future trends include:
1. Advances in AI-Assisted Annotation
With the rise of AI, we are witnessing new tools that employ machine learning to assist in the annotation process. These tools not only streamline the process but also increase accuracy by learning from previously labeled data.
2. Continuous Learning Systems
Future labeling tools may implement continuous learning systems that allow models to learn and improve from new data without needing extensive retraining, making the data annotation process more dynamic.
3. Integration with Cloud Services
As organizations increasingly adopt cloud-based solutions, labeling tools will likely integrate seamlessly with cloud services, enabling easier access and collaboration across distributed teams.
4. Enhanced User Interfaces
Future tools will focus on providing even more user-friendly interfaces, possibly with features like drag-and-drop functionality and real-time collaboration capabilities.
Conclusion
The effective implementation of labeling tools for machine learning can dramatically impact the success of AI and ML initiatives across industries. By selecting the right tools, leveraging platforms like keylabs.ai, and staying abreast of trends and challenges, organizations can ensure that they harness the full potential of their data. Don't underestimate the importance of high-quality, well-annotated data in your machine learning projects—it's the key to unlocking valuable insights and driving innovation.